short story
.png)
Hi,
My economics-oriented 2nd Ph.D. research is focused on the design-driven data servitization process, which binds together data products (raw material for services), data as a service (Daas) and data strategy in companies. It also includes data portfolio and lifecycle concepts.
See a more detailed research plan or continue reading.
LATEST NEWS
Goal is a practical toolkit
My intention is to define the Data as a Service tools which can be used in igniting, leading developing business driven data monetization in companies. Development will take advantage of Design Science Research methodology. You can expect lots of workshops and use case evaluations of the models discovered.
Data Monetization as the playground
Data monetization can be seen to include enhanced decision-making support through a better, more effective use of data; creating a unified understanding of data and a harmonised view of all relevant data; creating conditions for data-driven work and thereby becoming a digital company; increasing process efficiency; and building common objectives, benefit concepts and mindsets when dealing with data.
Data as a Product was one step towards servitized data. Open data started the tsunami of data and after the hype data monetization and commercialization have raised the often discussed topics also in the academic research (Gelhaar and Otto 2020; Kaiser et al. 2019; Moro Visconti et al. 2018). Yet the focus has been mostly in big data and technical aspects of the data. Emerging data economy markets are not fully yet here yet. Instead, we are still in the phase of learning to utilize and monetize data - both require data value streams which are expected to follow the service-driven paradigm more than goods-dominant paradigm.
Focus in data as a service
Focus in the research is in data as a service which are sold as commodities. Data product content is raw data or results of analysis process which might include AI or not. The content of data products is wrapped around with product element such as pricing plans, conditions, versioning, attractive name and description to mention a few. One of the fundamental aims is to define data product as accurately as possible. The added value is in the data which is packaged into easy to understand, buy and consume data products.
Data Product Toolkit is the first step towards the final framework
Data owners lack tools and skills to design data products. Furthermore, before data products can be built on top of a platform, those need to be designed. Platform might even offer testbed or simulation tools to test data products before production level publication. Initial set of business people oriented canvases have already been development as Data Product Toolkit, which offers canvases and other tools to design and manage data products, product lifecycle and product portfolios. This toolkit is now expanded to include ecosystem and operational excellence approaches, and focus is transferred from products to services (Daas)
Data Products, Data as a Service and Platforms
Data is increasingly not purchased as a batch or a dataset. Instead data consumer is purchasing access to data. One reason for this is the success story of subscription economy. We’ve seen this with Netflix and Spotify, but also in getting access to cars and other commodities instead of buying one. The purchased data product is stream of data which is either retrieved on customer request or pushed to customer upon changes in content. This resembles more a service than product in the pure format. More often “data product” is consumed via productized APIs. Consumers are not buying APIs. APIs provide modern access to discover, purchase and consume given data products. Thus I'm also prone to deal data as a service rather than products which are owned.
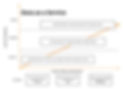
Data as Service dominates the emerging data economy. Read more about the claim from separate article and
Currently, the only scalable option to deliver data product content to consumers (apps) are APIs. Just as data has to be productized, also necessary APIs must be productized. Commonly data owners lack skills to productize APIs, market place to sell goods in. Thus we need a third-party - platform, which will offer productized APIs to access productized data products with modern plans including subscription.
Research questions
Research is always a journey. Doctoral dissertation related research even more. It is relatively easy to define framework for the research and direction where it is heading. Research questions are connected to the the research methodology as well and in some cases it may precede construction of the conceptual frame- work of study. A good research question is feasible, interesting, novel, ethical and relevant. Three research questions have been defined:
-
Q1: How to define Data as a Service and what are the characteristics of it?
-
Q2: What is the Data as a Service eXperience?
-
Q3: What is the process and needed tools to design Data as a Service commodities with lean and design driven approach?
-
Q4: How to manage the resulting data product/service portfolio?
Become coauthor
Below you'll find my planned articles to be written. I am more than happy to have coauthors since that enables more fluent authoring experience, multiple viewpoints, fresh ideas, fruitfull discussions and other benefits. I use Overleaf in the writing and that is not negotiable. Take a look at the article candidates and contact me if you want to participate in the research.
Below you'll find a link to more detailed research plan as well
Planned and ready articles
#1 Open DAta ProDUCT SPECIFICATION
Description
Data is increasingly also becoming an article of trade or commerce and approached with a product mindset. The process and tools to create and publish data commodities in data marketplaces are based on scattered and provider-specific metadata models. Data consumers also find it hard to compare data products and reusable development of dataops software solutions is cumbersome. Hence, we propose an Open Data Product Specification which is a vendor-neutral, open-source machine-readable data product metadata model, which enables interoperability between organizations, data platforms, marketplaces, and tools. The specification is built on experiences gained from over 30 data product cases. Our artifact can be used by practitioners to increase the speed of designing, testing, implementation, and deployment of data products, and to speed up emerging data markets development.
Coauthors
None - one man show!
Status
Accepted and to be published 2022. Prototypes Track.
Publication/conference
17th International Conference on Design Science Research in Information Systems and Technology
#2 DATA (developer) EXPERIENCE
Description
Data is servitized more and more. Just like any service, data as a service also has experience - we call it data experience. I don't yet what it is, but the concept started to occupy my head lately. Current customers of data offerings are technically savvy data analysts and in that sense, we might define data experience from a developer perspective much like with APIs. On the other hand are the customers of data as a service just developer-ish people? Probably not. The rising low-code/no-code usage among business people might be the other customer segment to consider. How are these two different? How do the expectations overlap and differ?
Services are created for customers and sales. That applies to data as a service as well. Data Product toolkit (don't let the name fool you!) helps you to focus on defining the value proposition for your customer and think about who is the primary customer. You must have a target persona, a stereotypical customer for whom you are offering data as a service. That persona can initially be fictional, but later on, you should aim for data-driven customer personas.
80% of the customers are data scientists
Different data services might have a slightly different customer profile, but in 80% of the cases, the customer is a data scientist. Keep in mind that traditional application developers are data as a service consumers too as well as their managers. Thus your service-related material and communication must serve both technically savvy and business-oriented people. At the moment data scientists are the sweet spot as the customers for data services.
Data scientists are a new breed of analytical data expert who have the technical skills to solve complex problems – and the curiosity to explore what problems need to be solved. They're part mathematician, part computer scientist and part trend-spotter.
Support customer’s existing tool stack
This in something you should keep in mind while drafting data service offering plans. You need to support the stack consumers are already using. You need to choose which tools and environments your customer needs to support out of the box. Luckily Data Product Toolkit forces you to consider data output channels thoroughly.
If your data scientist customers are hard-core PowerBI users, then you must optimize data services for that platform. If they want to use your data in Unreal game engines in order to create XR applications for the industry, then make sure data service is found by the Unreal game engine users. You get the picture, right? You should not expect costumers to adapt new tools just to utilize your data services.
Supporting tools and platform used by the customers, reduces the friction to become your customer. It lowers also the barrier and learning curve. Keep in mind that not all customers want to fiddle with huge code stack, but prefer to use low-code or even no-code platforms. Keeping your eyes on the instant usage of data services is also building capabilities for the future needs.
Quick results with minimal code development
Application programming interfaces also known as APIs are common technical solution to offer access to data products. Even if the stereotypical customer is technologically talented, you should keep in mind that not all want to use APIs and write code to consume your data products. That is because they might not have time, they just need quickly build proof of concept or use existing graphical user interface driven platforms and frameworks. In those cases you need to consider nocode and lowcode platforms as the most likely usage environment for your data products.
Data as a service customer profile is changing
Future is changing the setting for data services too. To be able to use data services in own apps or environments in minutes without writing any code is becoming the winning feature. Why? Currently we are witnessing lack of developers around the world. Despite of the efforts like training more developers, the situation is not expected to get much better. We will have shortage of software developers in the future. The same is happening with the data scientists as well.
The remedy is to drop the barrier to use data by other people than just hard core developers or data scientist. We - the average business developers - are in the spotlight soon. We are expected to build simple apps and consume data services to create value internally and externally by increasing sales, customer experience and efficiency.
We business people must become “DIY data scientists”. We must be able to use ready-made tools and data services to create quick and dirty solutions for business needs. Thus no-code and low-code solutions are deemed to rise as is expected to happen on the more traditional application development where APIs now reign.
Business oriented segment becomes more important
As I mentioned in the beginning you have two personas among the customers - technically oriented developers and business oriented managers. Nowadays the developer persona is in the focus. It seems that in the future you might want to focus more on the managers since they are destined to be your data product consumers. The size of the markets is also bigger among the not-so-technical business people.
Status
In progress. This is drafted and requires some more work on theoretical background and polishing. Overleaf link: https://www.overleaf.com/read/qycfwwjrttrj
Coauthors
Antti Loukiala
Intended publication/conference
Not known yet
#3 Framing the Data VALUE co-creation Process - A Model to Understand and Facilitate The Servitization Journey
Description
Companies are doing servitization as they transit from goods-dominant to service-dominant offering. Existing servitization journey frameworks are used and utilized in the research. Companies are already doing digitalization transformation journeys. Entering data monetization is also a journey (and possibly part of overall digital transformation) that often is ignited after finalizing the data strategy. However, companies do not have a clear model on how to proceed or understanding what the journey requires from their organization and what are the typical phases. By doing a series of case studies from various companies a framework is constructed.
Status
Draft, possibly a position paper?
Coauthors
-
Intended publication/conference
Not known yet
#4 Draw me a Data SERVICE
Description
What is a data as a service? How it has been discussed in the research literature (barely!). Data in raw format can be utilized internally with some effort. Situation changes when you want to sell data to others. Data must have clear pricing, solve a problem, offer value and ease of use. Data as a Service packages your data in the sellable format with business strategy fitting business plan. This is how I define data product at the moment. But what does the empiria have to say into this? Lets ask the people creating and consuming data products and data as a service!
Do a semistructured interviews among Platform of Trust / other community and discover the common elements of data products/services in the heads of owners (5-10) and consumers (5-10). Compare the two aspects to find out similarities and differences. Benefits marketplace designers as well as data product designers.
Coauthors
No
Status
in progress
Intended publication/conference
California Management Review (jufo 2)
#5 Rapid Design Driven data As a Service development
Description
I will do 4-5 case workshops with companies with Data Product Toolkit. I will interview participants before and after the workshops about the process of developing data products. At the same time I will improve the Data Product Toolkit and release the next version of it. In the interviews I will focus on the process, not the usability of the toolkit. Amount of interviews is around 10-15. Based on the results I can draft common framework for data product development process. Hypothesis is that will be cyclic and contain multiple steps. Does it follow the classic double diamond model or not? What are the differences compared to design process of a common digital product or service such as an API or app. Do the product designers wish to have multiple designs to select from which to proceed with? What are the steps they take from 0 to product concept testing?
Information gathered in this research can be used in data product tools development. Those tools are now needed more and more.
Status
Idea
Coauthors
-
Status
Idea
Intended publication/conference
Not known yet