While I’ve been discussing data economy with multiple customers, pricing has been one of the pain points. Also the understanding of the difference between data as a product and data as a service.
I stumbled upon a marvelous article about Value-Based Selling written by Keränen et al and published in the MIT Sloan Management Review. When I was reading the article I noticed that value is the word is that binds us together. I’ve been evangelizing the bigger change in business from Goods-Dominant Model to the Service-Dominant model. We’ve all heard dozens of times the examples of Netflix and Rolls Royce. Netflix changed the way we consume movies - took us from buying physical DVDs to buying access to stream of movies and series. DVD was a product, Netflix is a service. Movie rental was servitized. The Roll Royce converted jet engine business by servitizing the jet engines. Instead of selling the products (2 ton engines) they started to sell flying hours.
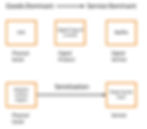
In the data economy, various professionals evangelize the idea that you should apply product thinking to the data. To me, that sounds partially right, but also partially outdated. Why? Applying 20 years old principles to the data economy is not sufficient nor wise. We most likely need to productize data, but that is just a step in increasing the value of data eg creating value we can sell. Adding the data servitization to the game changes the setting completely. Rolls Royce as an organization had to change dramatically when they decided to servitize jet engines. Becoming Service-Logic driven data commodity offering company requires different kinds of settings and skills as well as resources than just productizing the data.
In data economy, you still need to build the product just like Rolls Royce needs to build the engines (productizement). but also the wrapping service layer (servitization). If we really simplify things a lot, we can say that the simplest example of servitizing data products is to add modern well-documented, and maintained web API in front of it with code examples on how to use it and all the other developer experience related services. In short, we add value in the commodity. We'll return to the value part later in more details.
Data productizement and servitization
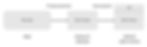
In productizement the need for tacit knowledge is removed or at least minimized. The content is standardized as far as needed and possible. The resulting data content is often a subset or result of an analysis derived from the raw data. One option is to package raw data and offer it in servitized format (via API) to partners, but not for 3rd party consumers. I’ve come to the conclusion that above path in data refinement is quite common since it enables two step monetization. You can monetize the data products for partners (and protect your own interests with limitations in terms and conditions). Then you can use the same productized data in servitizing the data for example as a visual tool sold as SaaS to the big mass.
Now let's return to the article written by Keränen. It presents different models to define your sales strategy by the value created, instead of the traditional product price which is often justified by multiple features.
The table below is a screenshot from the article and summarizes the article content pretty well. Which of the options should I take? Keränen et al suggest that you evaluate can you deliver quantified monetary value by selling better products, better process efficiencies, or guaranteed performance outcomes?

My thinking and logic of Data as a Service is heavily influenced and driven by Service-Dominant logic. And from that perspective, I see a lot of familiar elements in the above table. For example, the value focus looks very similar to Service-Dominant Logic. The same is for the Customer role. In the above table and in Service-Dominant Logic (SDL) customer is heavily involved always in value creation.
From data integration to dataless
Still, most of the data economy is still about datasets (Data Product) and “integration” of those sets to some larger data pool instead of consuming the data as a service via well-defined methods and digital value plumbing such as APIs. In other words, data is still copied and dumped into big lakes of data and then queried with SQL. We still live in the world of Data Products. We are still purchasing the data (product) and not the access (service) or results.
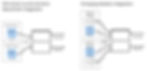
The above dualistic approach to data source consumption is discussed in more details in another post, but briefly, we live in the data integration world. Just like we did in the 1990s with code. Back then we reused code by integrating chunks of code together given that the attached open source license allowed us to do that. Then Web APIs appeared and changed the game totally. Now is the same with data. If you look at the data markets, you’ll find chunks of data packaged for sales. Those are datasets. On the other end of the rainbow is something I call dataless lakes. Of course, there is data as well, but it’s not stored in large quantities on the data platform. Instead what is added and indexed to the local data platform is the schemas of data streams and possibly some sample data.
Value-based Selling examples
Now after a long prelude, we can raise the question: what are examples of value-based selling in the data economy? Well, let’s see.
Product-centric view I’d say we are dealing with more or less pure form of data products. We are offering a set of data, which is said to contain for example up to date information about companies whatever is specific market segment. That set of information is gathered for you and you can do what ever you want with it. As a seller your arguments of sales are: optimized resources for value creation, the buyer can improve their customer experience by auto-filling forms for their customers. The bad news for sellers is that such lists can easily be imitated.
If we stick with the customer information topic example in the customer process-centric view, the most simple example is that we servitize the company information with an API. Then the buyer is not buying the dataset, but up to date information about a specific company on demand eg exactly when they need it. Delivery method is the current de facto digital plumbing - API. This clearly optimizes buyers' processes and data processing. They no longer need to download the data set and dump it into the database, instead, the buyer gets just the information they need. This data as a service can contain buyer-specific customization which ensures that the service is a perfect fit with the buyers' processes.
Third example is to provide data as a service commodity that analyzes the customer base and calculates predictions of the churn based on various factors. Most likely this requires access to various data sources on the buyer side. Value sold could be for example detecting customer churn changes in advance and providing (automatically) suggested actions to proactively improve the measured customer experience.